Next best action? How about next best everything?
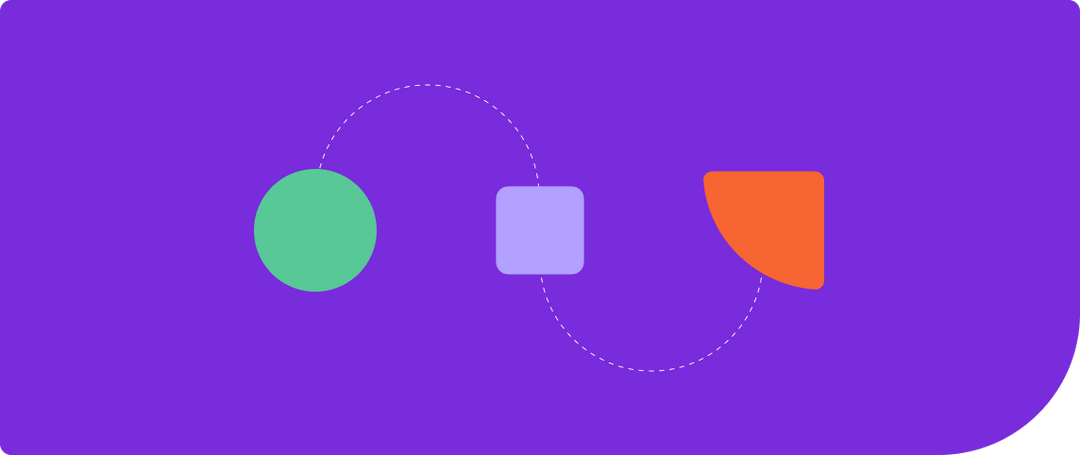
Why AI decisioning is next best everything
Marketing has always been awash in buzzwords, and two that have been buzzing the loudest lately are personalization
and AI
. Everyone and everything, it seems, is helping marketers personalize, no doubt with the help of AI. In this maelstrom of hype, marketers understandably are looking for simple explanations and clear value propositions.
One common method of using AI models to personalize are so-called next best action (NBA)
models. On its face, this seems like a simple idea – a next best action model should tell you the best action to take next with each customer. Who could say no to that? In practice, “next best action” is not a precise term, nor one used by data scientists or experts in machine learning. Many different models, tools, and capabilities bill themselves as “next best action.” Marketers need to understand what these models really do, and what kind of personalization they really offer.
How do we decide which action is best?
In principle, a NBA model could be extremely naive. For example, a bank could have a rule such as, “If a customer opens a checking account, offer a savings account. If a customer opens a savings account, offer a checking account.” This simple rule is of course not AI at all, and probably doesn’t deserve the name “next best action.”
In practice, marketers are using various types of machine learning (ML) to solve the next best action problem. Here are three approaches.
1. Predictive models
The most common approach to NBA is to implement a series of predictive models
, built with a type of machine learning called supervised learning
. For example, a company could deploy a model for each of its product lines which looks at historical data and predicts, given the customer profile and recent purchasing behavior, how likely they are to make their next purchase in category Y. These models can be combined to predict, for example, the top 3 products to recommend to each customer. This approach works best in industries like financial services, where the number of products or product lines is relatively small.
However, there is a fundamental limitation to this method. Suppose a model is correct that a customer is organically, based on historical data on similar customers, most likely to purchase product Y. That doesn’t mean that Y is the best product to promote! Perhaps we always show those customers product Y, and so they buy it, but some of those customers might happily buy higher-margin product Z. For example, a streaming or utility company might offer customers the next subscription tier, even though some customers would jump on “jump offers” which skip a tier. Since the company has historically not promoted those plans in those situations, customers haven’t historically bought them. So of course the predictive model has no way to know which customers have a propensity for the higher margin plan. In the language of ML, these models do not explore
and recommend options when the outcome is not yet known – they don’t try to experiment.
2. Collaborative filtering
The previous method is impractical for a retail company with thousands of products to promote, or a streaming company with thousands of movies and shows to recommend. In those industries, a common approach to NBA is a type of machine learning called collaborative filtering
. A streaming service, in picking which show to recommend to a viewer, has a sparse set of data – most viewers have not watched most shows. Collaborative filtering tries to find trends and patterns in this data to pick the shows the viewer hasn’t watched but is most likely to like. Similar models are used in ecommerce to recommend a product based on what a website visitor puts in their cart.
Collaborative filtering NBA methods have the same limitations as the propensity models approach – they are based on historical data, and don’t explore
to try and find options that might be better than the options that have worked in the past. As markets and customer behaviors change, the model will be slow to catch up.
3. AI decisioning
Unlike the previous two approaches, which make predictions solely based on historical data, AI decisioning
uses reinforcement learning
, a type of ML which experiments and learns. AI decisioning agents explore
the space of possible actions, trying out new options to discover what works best for each individual. Instead of simply doing what has worked best in the past, AI decisioning agents can experiment
and empirically discover
, for example, which customers are open to higher margin offers that the marketer may not have made to them in the past.
An AI Decisioning Engine, like the one that powers OfferFit
, experiments and learns using all available customer data. The AI agents learn and make recommendations based on hundreds of customer characteristics based on all available first-party data, giving recommendations that are truly 1:1.
When’s next? And what’s an action, anyway?
Suppose a marketer has chosen a model, perhaps one of the ones described above, for next best action. That begs the question: what’s an action? And for that matter, when is “next?” These are not pedantic questions – marketers have to make many decisions simultaneously when they engage with their customers.
When marketers speak of an “action,” they most often mean a product, promotion, or offer that they might make to a customer. A marketer’s model might tell her that the next best promotion to offer customer X is product Y. But that doesn’t tell the marketer all she needs to know. For example, what’s the right financial incentive? Perhaps the product has been discounted too much, and the customer would have happily converted with less of an incentive. And that’s not all – the NBA model doesn’t tell the marketer when to make the offer. In other words, when exactly is the “next” in “next best action? Should the marketer email today, or next week? In the morning, or in the afternoon? After all it hardly matters if we offer the right product, if the customer doesn’t read the email. Perhaps the customer is more likely to engage if the email had a different subject line or creative. For that matter, is it best to contact this customer by email in the first place as opposed to a text or a push notification? And if the customer ignores us, how long is it best to wait before contacting that particular customer again?
Clearly, simply knowing the next best product or offer is not enough. While we typically wouldn’t think of channel – or time of day, or day of the week, or the tone of a subject line – as actions
, these are decisions
that a marketer needs to make. It doesn’t do much good to say “We’re using next best action to personalize,” if the personalized offers are sent at the wrong time, at the wrong frequency, or over the wrong channel, or with the wrong incentive.
Marketers don’t simply need to find the next best action – they need to find the next best everything
.
Why AI decisioning is next best everything
AI decisioning like OfferFit’s, which is based on reinforcement learning, doesn’t simply find the next best product or offer for each customer. AI decisioning can find the best channel, time of day, day of the week, frequency, message, creative -- or indeed any other dimension a marketer wishes to test -- simultaneously. Moreover AI decisioning does not just do what's worked well in the past, but empirically discovers the best option 1:1 for each individual.
AI decisioning is not just next best action – it’s next best everything.
Nathaniel Rounds writes about AI and machine learning for nontechnical audiences. Before joining OfferFit, he spent 10 years designing and building SaaS products, with an emphasis on educational content and user research. He holds a PhD in mathematics from Stony Brook University.
Ready to start personalizing with AI decisioning?