Ready to build or buy machine learning? First ask these five questions
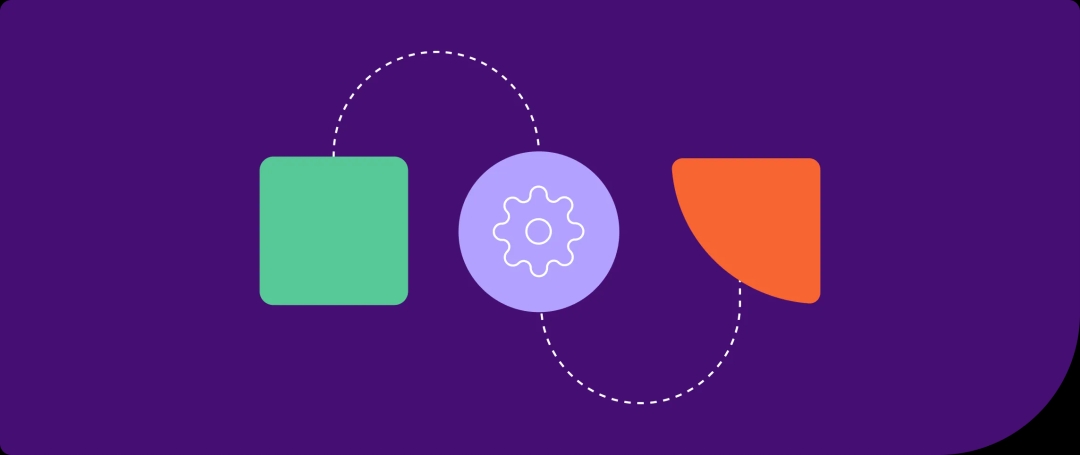
If everywhere you go, you hear buzz about machine learning (ML)
– well, you’re not alone. In 2022, NewVantage Partners reported that 97% of Fortune 1000 companies are investing in data initiatives, and 91% are investing in AI. And it seems that nowhere has this trend been more pronounced than in marketing. In recent years, marketers have used ML solutions to solve a staggering array of problems.
For example:
Churn prediction
– identify customers at the highest risk for churn,Customer segmentation
– find patterns in data to automate the process of creating customer segments,Website personalization
– choose a modal or landing page that will best engage each customer, andAI decisioning
– empirically discover optimal actions for each customer. (This is OfferFit’s focus.)
But when it comes to machine learning and data science, most enterprises can tell war stories of failed projects and abandoned solutions. How can marketers make sure machine learning implementations are a success rather than a cautionary tale? As they look to add ML capabilities to their martech stack, the question looms – do we build or buy
? In truth, products from an external vendor and tools built in-house should be subject to the same due diligence.
Before building or buying, ask these five questions
.
1. Do we have a clear goal?
The easiest way to fail is to start without a goal
. And let’s be clear – “we want a neural net,” doesn’t count. Marketers need to understand the business goal they hope their new investment will help them achieve. For example, a company building a churn prediction model should be able to quantify the reduction in churn they hope to see. A marketer might experiment to discover the best upsell offers for each customer – in that case, the success metric might be the average revenue per user.
Of course, a goal is only measurable if you have the data necessary to measure it.
Say a company uses ML to customize messages to engage customers in a loyalty program. To know if the campaign is effective, they’ll need to be able to connect customer actions, for example on their website, to customer engagement in their loyalty program.
With a clear goal, and a clear and measurable success metric, marketers are set up to evaluate the ROI of any solution they build or buy.
2. Do we understand our costs?
In measuring benefits, don’t lose sight of costs. It might seem easy to measure costs of an external solution – you pay a price for a product. But marketers shouldn’t overlook the need to integrate the new solution into an existing tech stack, or training costs for the team that will use the product. The right vendor should be able to articulate these costs.
The costs of building in-house can be even more insidious.
Typically, a company’s scarcest resource is their team’s time. Marketers need to understand the internal time and resources a project will take before committing to building. And what will happen when data science and engineering teams have moved on to other projects? Marketers need a plan to maintain their ML solutions over time.
It might seem easier to integrate a solution built in-house into a martech stack. But every enterprise is already using many 3rd-party products, such as a data warehouse, customer data platform, and activation system. If a company builds a new ML solution, their data science and engineering teams will also need to spend time integrating that solution with the rest of their tech stack. A 3rd party vendor likely already has experience working with other major platforms. Of course, marketers should diligence this capability in any vendor they work with.
3. Do we have the necessary expertise?
To build in-house, an organization needs the right background and expertise.
Different ML solutions use different underlying technologies. Machine learning is usually classified into 3 types:
Supervised learning
: predicting an outcome based on historical data.Unsupervised learning
: identifying patterns in data.Reinforcement learning,
orAI decisioning
: experimentally discovering optimal actions. (This area is OfferFit’s focus.)
In-house data science teams typically have experience building models based on supervised and unsupervised learning, such as churn prediction or cluster analysis. These types of machine learning models are well supported by “off the shelf” tools, and can be stood up relatively quickly.
Reinforcement learning agents, such as those that power OfferFit's AI Decisioning Engine, are a different story.
Reinforcement learning is a relatively new and specialized field of machine learning. Companies should be sure they have the necessary expertise in-house before deciding to build.
4. What is our time to value?
Say a marketer has a clear, measurable goal, a grip on costs, and confidence their in-house data science team or their chosen vendor has the necessary expertise. Now what? Companies investing in ML need to understand how long it will take to see returns – their time to value
.
Always ask a vendor how long it typically takes them to go live. When companies build in-house, time to value could be much murkier. Standing up an engineering and data science team is expensive, and their time is scarce and valuable. What other priorities might come up that could push back the schedule? The longer an ML project goes on without demonstrating value, the likelier it becomes that someone decides it’s time to pull the plug.
5. How will we keep pace with new innovations?
Machine learning is a rapidly developing field, with new techniques and tools emerging every year. Companies that build a solution in-house should ask themselves, “Will we be able to retrofit in response to technological innovations?” If not, they might soon be looking to implement a new solution, and asking themselves if they should build or buy all over again.
Ready to start personalizing with AI decisioning?